mlpack
blog
|
Table of Contents
A list of all recent posts this page contains.
- Visualization Tool - Summary
- Visualization Tool - Week 08-11
- Visualization Tool - Week 06-07
- Visualization Tool - Week 05
- Visualization Tool - Week 04
- Visualization Tool - Week 03
- Visualization Tool - Week 02
- Visualization Tool - Week 01
- Visualization Tool - Community Bonding Period
- Implementing Essential Deep Learning Modules - Summary
- Implementing an mlpack-Tensorflow Translator - Summary
- Advanced Kernel Density Estimation Improvements - Summary
Visualization Tool - Summary
This post summarize my work for GSoC 2020
Overview
The proposal for Visualization tool included the implementation of class which would be able to log metrics and a callback function that can be passed to ensmallen optimizer to log metrics.
Implementation
A separate repo was created to undertake the project to avoid adding dependencies to mlpack main package.
Initial Setup PR#1
- The implementation started with setting up the repo structure and making it compatible to be installed over any os.
FileWriter
class andSharedQueue
class was implemented in this PR.
Setup CI pipeline PR#3
- Then we setup the pipeline for azure builds so that we continously know that we are not breaking anything.
Setup Catch Testing PR#5
- This PR was raised to add Catch2 as testing framework and write some test.
Add Image Support PR#6
- We added image support in this PR.
Add Text Support PR#7
- We added text support in this PR.
Add PRCurve Support PR#12
- We added PRCurve support in this PR.
Add Embedding Summary Support PR#10
- We added embedding support in this PR.
Add Histogram Support PR#13
- We added Histogram support in this PR.
Add Callback Support PR#14
- We added Callback support in this PR.
Post GSoC
Improve cmake scripts a litle bit, possible improvements in implementation and Improve callback for bettery visualization.
Acknowledgement
A big thanks to Toshal, Brim, Rcurtin, Zoq and the whole mlpack
community. This was my second GSoC with mlpack, and I am happy that once again I was successful in it. I gathered a lot of knowledge in these past 3 months. I will continue to be in touch with the mlpack
community and seek to do more contributions to the project in the future.
Also, I think its time to order some mlpack stickers :)
Thanks :)
You can find my weekly reports here
Thanks, Signing off :)
Visualization Tool - Week 08 - 11
Sorry for being late in updating the blogs, but this Post would contain updates from Week 08 through 11, So let's start.
Over the week we were able to merge two PRs, the first one was text-support and then prcurver-support.
Also we have a pr for embedding-support, ready to be merged and hope full would be merge this week.
Two fresh PR's were raised, the first one was for histogram-support and then mlbaord_logger-callback. The callback is the last agenda on our list before the program ends.
Lastly before the program ends we had some stretch goals, we would like to acheive that too. A bit of cleaning also needs to be done such as improving cmake, there are some issues raised about it such as https://github.com/mlpack/mlboard/issues/14 and https://github.com/mlpack/mlboard/issues/8.
Thank you :)
Visualization Tool - Week 06 - 07
Sorry for being late in updating the blogs, but this Post would contain updates from Week 06 and 07, So let's start.
So the image-support-pr was merged, and last week two new pr was raised which was to add support for embedding-summary and also prcurve-summary. Hopefully, we would be able to merge all the three PRs including the PR for text-summary.
In the upcoming week, I would like to debug an error in embedding-support pr and would target adding histogram summary. So My target would be two add all the support before entering in the third phase.
And in the third phase, I would focus on writing a ensmallen callback to log the data and then all clean up if there are any and improve testing if coverage if possible.
Thank you :)
Visualization Tool - Week 05
Hi, Sorry for being late to update you all, This week didn't go well for me Since I took 3 days off due to some medical condition. The first half of the week went as expected, and we were able to merge the ci-windows PR successfully and also attempts were made to improve the image and text support pr.
In the last meeting we decided to drop the plan of supporting audio summary and focus on the histogram, and This week I would be devoting my time to implement support for plotting histogram.
Also a piece of good news, I successfully passed the first evaluation. That's it from this week, will keep you all updated with the progress of mlboard
, till then bye :)
Visualization Tool - Week 04
Hi, Welcome to another blog, This week was less of coding and more of research, I did go through the implementation of logging histogram summary and pr-curve summary and also text summary. And now I am more than familiar with them and would implement them over the next two weeks.
Also last week, I implement support for logging text summary, you can view the pr here, It needs to be reviewed and polished. The Catch-testing PR was merged after some hiccups of mkdir()
and rmdir()
function which caused a problem in the different operating systems. And Also some polishing was done in Image-support pr after some small reviews.
Also, an attempt was made here to set up CI workflow for windows operating system for the repo, The dependencies were installed and configured correctly but for some reasons mlboard build failed. I am not the best person to know about windows os and haven't used for almost 3 years and hence I would take some time to look into it and debug it. But parallelly I would work on adding logging support for other summary types.
That's it for this blog, Will keep you updated with the future progress. Till then Bye.
Visualization Tool - Week 03
Hi, Welcome to the blog for the third week :)
This was one of the toughest week for me since the start of the GSoC program, Since I had to debug some cmake and protobuf issue. After numerous downloads and install of protobuf library, I was finally able to debug it. And hence catch testing has been finally setup up. Now I can focus on writing tests over the next week. Thanks to and for the help :).
Also, Support for logging of the image was added in a new PR#6. So both of the goals were accomplished which were set during the previous blog.
If you want to try the new Image Logging API for arma::mat
, you can use the following snippet of code.
Note : Please download the csv from here
The outuput would be
It took around 4 sec to log 50 Images, and IMO that is really good. Also, To log 42000 images it took 67.6837s.
The ouput would be similar to
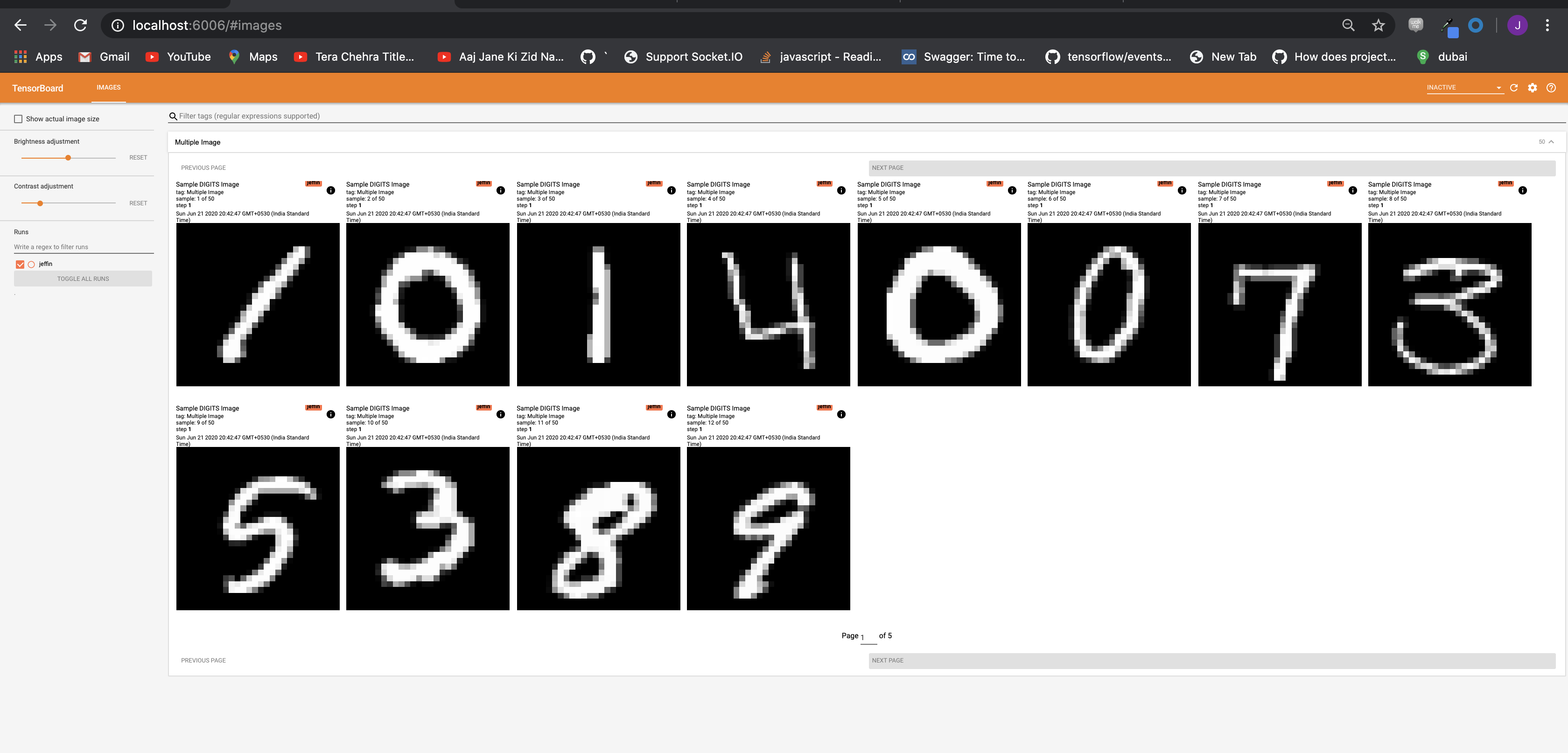
Next week is the final week before the first phase ends, and therefore I would like to completely get rid of both the PR ( Image support and catch-testing ), and then focus on logging text, audio, histogram during the second phase. Till then Bye :)
Visualization Tool - Week 02
The second week of GSOC concluded successfully. During the week I resolved the comments made during multiple reviews on the first PR, and now it has finally been approved and ready to be merged. So we do have our first look of mlboard
ready.
Also during the week two separate PR#3 and PR#4 were raised to set up the azure pipelines for the CI jobs.
Also during the weekly meeting, it was decided to set up some unit testing for mlboard, and we found catch2
to be interesting and easy to be adopted since it is a header-only library. A draft PR#5 was raised to be able to integrate catch2
into mlboard
for testing using CMake
.
Going ahead I plan to complete the cache unit testing work and also add support for Logging Image summary using mlboard. And for both, I am assuming two weeks of the expected time of implementation.
So that was the whole update from the past week and also plan for upcoming weeks, Will keep you all posted. Thanks for reading it through. Good Day.
Visualization Tool - Week 01
Hi, So the first week of coding period ended and we are on time as of our timeline. The implemenation of Sharedqueue, filewriter and Summary class for plotting scaler was completed. Also work with cmake was partially completed, it may not be the most robust one but certainly works for now.
The docs were updated and you can see an example and also how to build https://github.com/jeffin143/mlboard/blob/cmake-setup/README.md "here"
My agenda this week would be to add support for histogram, for that I may need to write an helper function similar to numpy.histogram
and also another one would be to study more about cmake and build system down the lane. In short it would be my agenda to make or improve the cmake script during the whole gsoc period. I don't want it to be a blocker and hence I just came up with a simple one and iteratively we could improve it.
helped in setting up the linting job, and the first pr#1 with the cmake and other class implemenation was raised and are in queue for a review. I am guessing it would be merged soon, and then we can start with histogram.
We still do have meetings reguarly on IRC every wednesday and sunday @ 10:30 IST, you could read the logs to track the development or could join and suggest any improvements if you do have
Thanks for reading till the end :). Will meet you in the second blog. Till then BYE.
Visualization Tool - Community Bonding Period
Hi eveyone, Glad to tell that I was lucky enough to be accepted second time as gsoc student for the same org :). And this time I would be working to come up with a Visualization tool
for mlpack. This time my mentors would be Ryan Birmingham
know by github handle as @brim
and Toshal Agarwal known by github handle as @walragatver
.
During the community Bonding period we had several meetings to discuss about the high level desgin for the tool and we cam up with the following :
The initial proposal is intended for enabling users to visualize Mlpack data using the TensorFlow's TensorBoard. The plan of this summer is to develop a logging tool for users to log data in the format that the TensorBoard can render in browsers. Thereby leavraging the power of tensorbaord's frontend and just focusing on the logging part. The typical workflow is as follows :
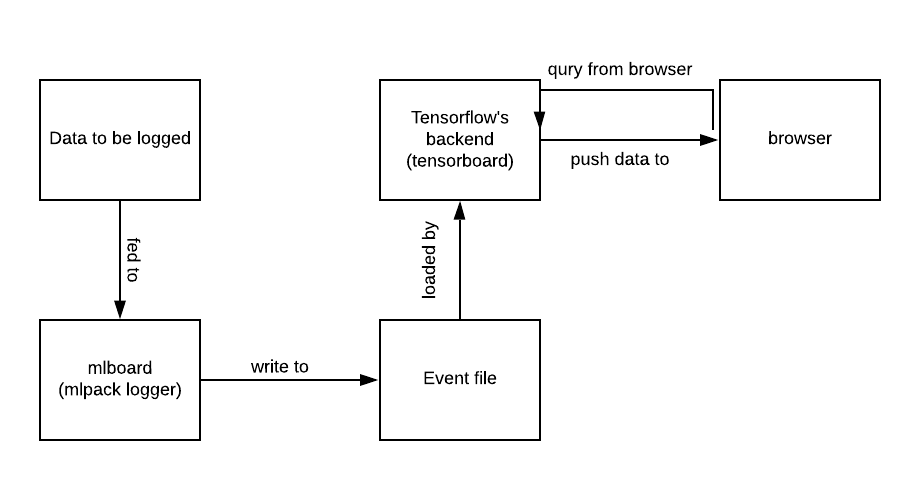
Asynchronized logger
: The logger would be implemented asynchronously so that training of model or any other operation doesn't get effected.
High Level Design :
We plan to add most of the data types that are aldeardy supported in tensorboard : scaler
, image
, audio
, text
, histogram
, embedding
and pr-curve
.
class Summary
: This class would be responsible to hold the data such as scaler summary or image summary and convert it to event and then push it the queue through the filewrite class.
class FileWriter
: This class is reponsible for writing events into the file through the logger thread.
class SharebaleQueue
: This class is responsible to maintain a queue which could be thread safe and shared among the main thread (summary class) and logger thread (filewrite class)
The way whole workflow works is
- The user creates a
FileWriter
object instance by providing the constructor with a path representing the location where data is going to be logged. For example:fw = FileWriter(logdir="./logs")
- Then a user can call the corresponding
summary
API to push the data to be logged into the event queue. For example, `summary.add_scaler('test',1,13, fw). The arguments could be any depending upon the type of scaler along with the filewrite object. This filewriter internally pushes the event into shared queue.
Parallely
writeEvent()` which is a logger thread access the shared queue and pops out element and then logs it into event file
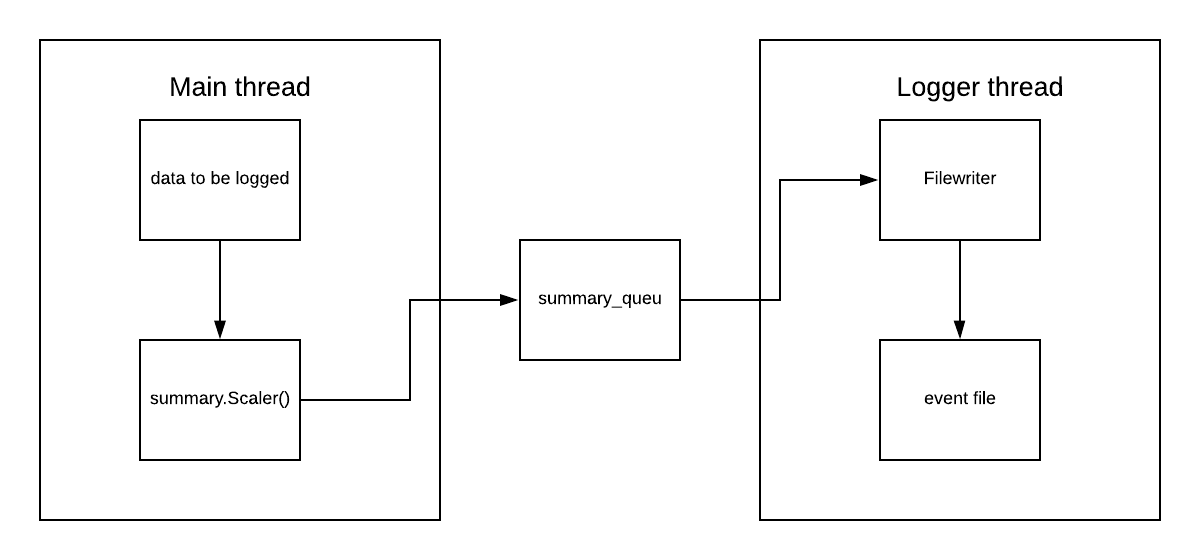
Acknowledgements and References :
Special thanks to @RustingSword
, author of RustingSword/tensorboard_logger
, who provided insightful ideas and hours of discussion to help me understand the backened thoroghly. Also some of the refrences are taken from the following repos. We would like to thank them for their good work :)
- https://github.com/dmlc/tensorboard
- https://github.com/TeamHG-Memex/tensorboard_logger
- https://github.com/lanpa/tensorboard-pytorch
- https://github.com/reminisce/tensorboard-mxnet-logger
All of the above repos helped us to come up with design and also made it possible to understand how to log exactly independent of TensorFlow. And also gave us enough insights about the internal of tensorboard and tensorflow logging class.
Implementing Essential Deep Learning Modules - Summary
Overview
This summer I got an opportunity to work with mlpack
organisation. My proposal for Implementing Essential Deep Learning Modules
got selected, and under the mentorship of Shikhar Jaiswal
and Marcus Edel
I was working on it for the last three months.
The main aim of my project was to enhance the existing Generative Adversarial Network (GANS)
framework present in mlpack
. The target was to add some more functionality
in GANs
and to implement some new algorithms to mlpack
such that performance of GANs
is improved. The project also focussed on adding some new algorithms so that testing of GANs
becomes feasible.
Implementation
Improving Serialization of GANs
The first challenge in the existing GAN
framework was to enable loading and saving the GAN
model correctly. In mlpack
the loading and saving of models was done with the help of Boost Serialization
. The most important responsibility was to have complete consistency so that all the functionalities of the model are working perfectly fine after saving and loading the model. The Pull Request #1770 focussed on this and it will get merged soon.
Providing Option to Calculate Exact Objective
In order to make Dual Optimizer functionality efficient it was required to remove the overhead of calculating the objective over the entire data after optimization. In order to do so I opened #109 in mlpack's ensmallen
repository. It is merged and now ensmallen's Decomposable
Optimizer's provide an option to user that weather he wish to calculate exact objective after optimization or not.
Dual Optimizer for GANs
Various research papers published related to GANs
used two seperate Optimizer's in their experiments. However mlpack GAN
framework had only one optimizer. Due to this testing of GAN
was quite tedious. So the main aim of the #1888 Pull Request was to add Dual Optimizer
functonality in GANs. The implementation of Dual Optimizer
is quite complete and currently it's testing is going on.
Label Smoothing in GANs
One sided label smoothing mentioned in the Improved GAN paper was seen to give better result while training GAN
model. Also in order to add LSGAN in mlpack label smoothing was required. It's implementation and testing is quite complete in #1915, however to make label smoothing work perfectly some commits from serialization PR were required. So, it will get merged once #1770 gets merged.
Bias Visitor
In order to prevent normalizing the Bias
parameter in Weight Norm Layer a bias visitor was required to set the bias parameters
of a layer. The first step was to add getter method Bias()
in some layers. Afterwards these getter methods were used to set the weights. The visitor is merged with the help of #1956 PR.
Work Around to add more layers
The Boost::variant
method is able to handle only 50 types. So inorder to add more layers to mlpack's ANN module a work around was required. After digging somewhat about the error online I found a work around. The Boost::variant
method provides an implicit conversion which enables adding as many layers as we can with the help of tree like structure. The #1978 PR was one of the fastest to get merged. I just completed it in two days such that the Weight Norm layer gets merged.
Weight Normalization Layer
Weight Normalization is a technique similar to Batch Normalization
which normalize the weights
of a layer rather than the activation. In this layer only Non-bias
weights are normalized. Due to normalization the gradients are projected away from the weight vector, thus testing the gradients
got tedious. The layer is implemented as a wrapper around another layer in #1920.
Inception Layer
In order to complete my Frechet Inception Distance PR GoogleNet was required. In order to do that Inception Layer
is required. There are various versions of the Inception Layer
. The first version of the layer is quite complete. However #1950 will be merged after implementing it's all three versions. The Inception Layer
is basically just a wrapper
around a Concat
Layer.
Frechet Inception Distance
Frechet Inception Distance
is used for testing the performance of the GAN
model. It uses the concept of Frechet Distance
which compares two Gaussian
Distribution as well as the parameters of the Inception
model. In order to get the parameters of Inception model #1950 will be required to merge first. The Frechet Distance
is currently implemented in #1939 and it will be integrated with Inception Model
once it is merged.
Fix failing radical test
While working on this PR I learned how important and tough testing is. The RadicalMainTest
was failing about 20 times in 100000 iterations. After quite a lot of digging it was found that the reason was that random values were being used for testing. With this PR I learned about Eigen Vectors
, Whitening
of a matrix and many other important concepts. The #1924 PR provided a fix matrix for the test.
Serialization of glimpse, meanPooling and maxPooling layers
While working on Gan Serialization, I found that the glimpse
, meanPooling
and maxPooling
are not serialized properly. I fixed the serialization in #1905 PR. Finding the error was one of the patience testing job but it felt quite satisfied after fixing it.
Generator Update of GANS.
While testing GANs
I found one error in the update mechanism of it's Generator
. The issue is being discussed with the mentors, however the error seems ambiguous. Hence, #1933 will get merged after arriving at the conclusion on the issue.
LSGAN
Least Squares GAN
uses Least Squares
error along with smoothed labels
for training. It's implementation is quite complete and #1972 will get merged once LSGANs testing will get completed.
Pull Request Summary
Merged Pull Requests
- Pull Request ensmallen/#109 - Providing option to calculate exact objective
- Pull Request #1905 - Serialization of glimpse, meanPooling and maxPooling layer
- Pull Request #1920 - Weight Normalization Layer
- Pull Request #1924 - Fix failing radical test
- Pull Request #1956 - Bias Visitor
- Pull Request #1978 - Work Around to add more layers
Open Pull Requests
- Pull Request #1770 - Improving Serialization of GANs
- Pull Request #1888 - Dual Optimizer for GANs
- Pull Request #1915 - Label Smoothing in GANs
- Pull Request #1933 - Error in Generator Update of GANs
- Pull Request #1939 - Frechet Inception Distance
- Pull Request #1950 - Inception Layer
- Pull Request #1972 - LSGAN
Future Work
- Completion of Open Pull Requests.
- Addition of Stacked-GAN in
mlpack
. - Command Line Interface for training
GAN
models. - Command Line Interface for testing
GAN
models.
Conclusion
I learned quite a lot while working with mlpack till now. When I joined mlpack I was quite a beginner in Machine Learning
and in the past months I have learned quite a lot. I also learned quite a lot how Object Oriented Programming
helps in Developing a big project
. Also my patience got tested while debugging
the code I have written. Overall it was quite good learning and fun.
I will keep contributing and will ensure that all of my Open PR's get merged.
I would also like to thank my mentors ShikharJ
, zoq
and rcurtin
for their constant support and guidance throughout the summer. I learned quite a lot of things from them. I would also like to thank Google to give me an opportunity to work with such highly experienced people.
Implementing an mlpack-Tensorflow Translator - Summary
The Idea
The general objective of this project is to allow the interconversion of trained neural network models among mlpack and all other popular frameworks. For this purpose, two converters have been created:
- ONNX-mlpack-converter
- mlpack-Torch-converter
ONNX being a central junction supporting a number of popular frameworks including but not limited to Tensorflow, Torch, Keras and Caffe, the Tensorflow-Torch-ONNX-mlpack conversion is made possible through this project.
The reason why we chose Torch over ONNX for the mlpack-Torch-converter is because the C++ implementation of the ONNX library doesn't directly support model creation. It can still be done though, as nothing is impossilbe to achieve and ONNX models are nothing but protobuf files. There was no robust reason of choosing Torch over Tensorflow except for the fact that Torch's C++ API seemed to be more robust. That being said it actually boils down to one's personal choice and it rightly did boil down to my preference of exploiting the opportunity of learning a new framework instead of working with Tensorflow, with which I was largely familiar.
The Code
The code directly associated with the converters are in the repository https://github.com/sreenikSS/mlpack-Tensorflow-Translator under the /src folder, while the tests are under the /src/Tests folder and converted models under the /Examples folder. The tests need and will receive an updateThis project mainly has three source files:
- model_parser.hpp which is a header file supporting the creation of an mlpack model from a user-defined json file
- model_parser_impl.hpp which contains the implementation of the definitions present in model_parser.hpp
- onnx_to_mlpack.hpp which contains the necessary functions to convert ONNX models to mlpack format
- mlpack_to_torch.hpp which contains the necessary function to convert mlpack models to Torch format
This project however, has additional dependencies like LibTorch and ONNX and is/will be clearly mentioned in the readme file. This is supposed to exist as a separate repository under mlpack.
JSON parser
This parser can be used in a number of ways, like obtaining a LayerTypes<>
object corresponding to a string containing the layer type and a map containing the attributes as follows:
It can also be used to convert a json file to an mlpack model by calling the convertModel()
function and if needed overriding the trainModel()
function. An example of the using the converter which will train the model and display the train and validation accuracies is:
The trainModel()
has not been overridden here but it may be necessary in most cases. However, it should be noted that most but not all layers, initialization types, loss functions and optimizers are supported by this converter. An example of a JSON file containing all the details can be specified as:
ONNX-mlpack converter
Given the ONNX model path and the desired path for storing the converted mlpack model, the converter can do the rest. However, for converting models that take images as input, i.e., convolutional models, the image width and height need to be mentioned too. An example of the usage is:
To be noted is that most but not all layers are till now supported by this converter.
mlpack-Torch converter
This converter provides an API similar to the previous one. An example would be:
In case the case of convolutional models too, the input image dimensions need not be mentioned. For directly obtaining the Torch model from a given mlpack model, the convert()
function can be used as shown below:
This converter also has some limitations pertaining to the layers that can be converted. Moreover, this converter is not yet in working state right now because of a number of yet to be merged PRs in the main mlpack repository.
Additional Pull Requests
The above converters did require a number of changes to the original mlpack repo and are listed as follows:
- 1985 adds accessor and modifier methods to a number of layers.
- 1958 originally meant to add the softmax layer to mlpack's ANN module but the PR itself is a bit messed up (has unwanted files associated with it) and needs to be pushed again.
There are also a couple of pull requests that require some rectification before I can push them.
Acknowledgements
I owe the completion of this project to the entire mlpack community for helping me out whenever I got stuck. My mentor Atharva had given me the exact guidance and support I required during this period. My concepts about backpropagation have been crystal clear after we manually wrote down the steps on paper. He used to give me hints to encourage me and in the end I could do it entirely by myself. Understanding this as well as mlpack's way of implementing them (the matrices g and gy in the Backward()
function were the confusing ones) took around an entire week but it was a fun experience. This is just one instance, there were many more during this 12 week period. Marcus and Ryan were also no less than pseudo-mentors for me.
Marcus was my go to person during the Summer for any doubt regarding the C++ implementation of the mlpack ANN implementation or pretty much anything else. I have a habit of spending too much time on things that seem difficult to solve, sometimes a couple of days (when I should have ideally tried for a couple of hours before asking for help) and even if I fail to solve it, I would ask Marcus on the IRC and we would arrive at a solution in less than an hour.
Ryan has been a constant source of support since my association with mlpack. When I had started with an issue, back sometime in February, Ryan had helped me design the layout of the program which would later be the JSON model parser. There were numerous other instances during this period (and many more to come) when my code wouldn't work and Ryan would help me solve it.
Last but not the least, I have also learnt a lot from the general discussions in IRC and would like to thank each and everyone in the mlpack community for the brilliant interaction. I would also like to thank Google for giving me this opportunity to get involved in open source and with the mlpack community in particular.
Advanced Kernel Density Estimation Improvements - Summary
Abstract
Kernel Density Estimation
(KDE) is a, widely used, non-parametric technique to estimate a probability density function. mlpack
already had an implementation of this technique and the goal of this project is to improve the existing codebase, making it faster and more flexible.
These improvements include:
- Improvements to the
KDE
space-partitioning trees algorithm. - Cases in which data dimensionality is high and distance computations are expensive.
Implementation
We can summarize the work in 3 PRs:
Implement probabilistic KDE error bounds
Up to this moment, it was possible to set an exact amount of absolute/relative error tolerance for each query point in the KDE
module. The algorithm would then try to accelerate as much as possible the computations making use of the error tolerance and space-partitioning trees.
Sometimes an exact error tolerance is not needed and it would mean a lot for flexibility to be able to select a fuzzy
error tolerance. The idea here is to select an amount of error tolerance that would have a certain probability of being accomplished (e.g. with a 95% probability, each query point will differ as much as 5% from the exact real value). This idea comes from this paper.
This is accomplished by probabilistically pruning tree branches. This probability is handled in a smart way so that when an exact prune is made or some points are exhaustively evaluated, the amount of probability that was not used is not lost, but rather reclaimed and used in later stages of the algorithm.
Other improvements and fixes were made in this PR:
- Statistics building order was fixed for cover and rectangular trees.
- Scoring function evaluation was fixed for octrees and binary trees.
- Simplification of metrics code.
- Assignment operator was added for some trees (issue #1957).
Subspace tree
The dominant cost in KDE
is metrics evaluation (i.e. distance between two points) and usually not all of these dimensions are very relevant. The idea here is to use Principal component analysis
(PCA), as a dimensionality reduction technique, to take points to a lower dimensional subspace where distance computations are computationally cheaper (this is done in this paper). At the same time the idea is to preserve the error tolerance, so that it is easy to know the amount of maximum error each estimation will have.
This is done by calculating a PCA
base for each leaf-node and then merging those bases as we climb to higher nodes.
This PR is still a work in progress.
Reclaim unused KDE error tolerance
This paper mentions the idea of reclaiming not used error tolerance when doing exact pruning. The idea is that, when a set of points are exhaustively evaluated, the error of these computations is zero, so there is an amount of error tolerance for pruning that has not been used and it could be used in later stages of the algorithm. This provides the algorithm with the capability of adjusting as much as possible to the error tolerance and pruning more nodes.
Thanks to Ryan
's derivations, we also realized that the bounds of the previous algorithm were quite loose, so a lot of error tolerance was being wasted. This has been reimplemented and will probably represent a huge source of speedup.
Future work
There are some ideas that we did not have time to finish but are quite interesting:
- Finish subspace tree PR.
- In the proposal there was the idea of implementing ASKIT and this is really interesting for me.
Conclusion
This has been an awesome summer. I had the opportunity to contribute to a meaningful project and will continue to do so in the future, since there are many ideas that came while I was working on this or did not have time to finish. It has been a very enriching experience for me, I learned lot, it was a ton of fun and, definitely, debugging skills got sharpened.
I would like to thank the whole mlpack community as well as Google for this opportunity. A special mention has to be made for my mentor rcurtin
, without his help when I was stuck and his new ideas I would not have enjoyed this as much, so thank you.
For people reading this and thinking about applying for GSoC in the future: Apply now, it will be fun and you will learn a lot from highly skilled people.